The Netflix Leadership Model
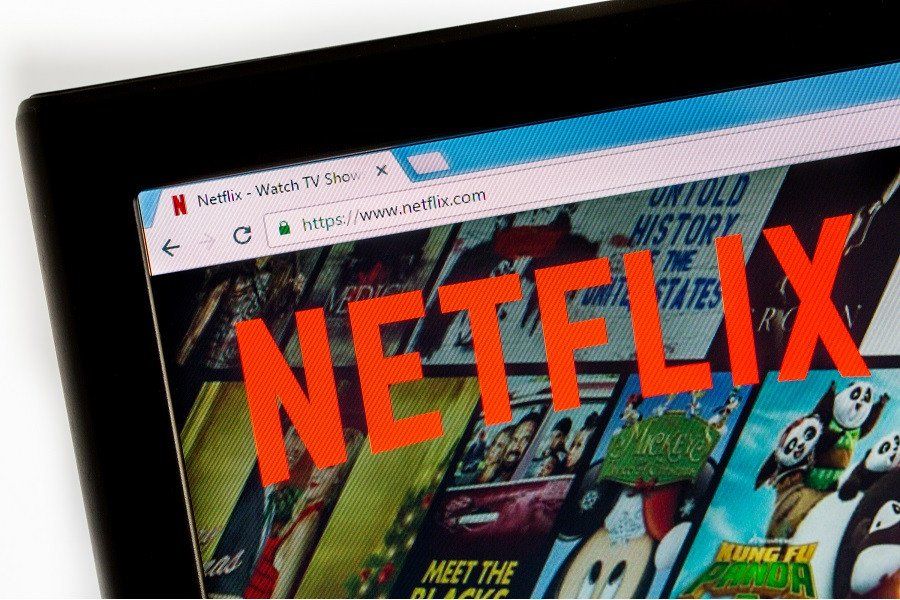
Understanding how the streaming service uses its offset data
EIGHTY per cent of the hours streamed on Netflix are of programmes prompted by its recommender system.
Like it or not, the streaming service has an uncanny ability to predict our viewing preferences and leave us unwittingly hooked on a range of shows we might never have encountered otherwise. Sitting at the core of its clever system is robust personalisation, and it is driven by machine learning.
What is an ‘offset’?
Since the forgotten days of mail order movies, Netflix has built a business model for the identification of patterns in our likes and dislikes.
Such is its ability to pinpoint our preferences. Netflix now counts as one of the world’s best organisations when it comes to the use of artificial intelligence for personalisation.
At the centre of this personalisation is a suggestion engine built upon sophisticated mathematics that calculates the conditional probability that something will happen. Alongside a series of complex interactions, the engine bases its predictions on viewer ratings and history. For instance, will someone like the movie ‘Top Gun’ because he had also given a high rating to ‘Rocky’ (my favourite movie)?
As the company explains: “We want to estimate the conditional probability that a subscriber will like a film, given his or her particular viewing history, in light of the ratings data from all other subscribers. This will allow us to personalise film recommendations for each viewer.”
As they point out, however, much of the data is missing as “most subscribers haven’t watched most films.”
To overcome this challenge, Netflix uses something called an offset – a gauge of how far something deviates from the average. In their world, some movies are more popular than others and therefore have a positive offset.
Similarly, some viewers are more cynical and rate movies more harshly, which gives them a negative offset.
Understanding this offset concept is critical in making a personalised recommendation. After all, an average is not unique.
The winners of the 2009 Netflix US$1 million challenge to improve the company’s prediction system by 10 per cent or more went beyond a viewer’s base rating. They highlighted the importance of understanding a rating in the context of other ratings and derived their predictions from some fundamental equations: overall average across all films + a particular film’s offset + the viewer’s specific offset + the user-film interaction.
A model for leadership
Today, Netflix uses this information to make better recommendations to subscribers, and you should be employing similar tactics every day when you lead.
Work is full of offsets. Some employees are faster, while others are slower. Some are highly experienced, others are novices. Some are more diligent, others flippant.
The list goes on and it applies to tasks as much as employees: some tasks are more complicated than others, some require teamwork while others can be tackled single-handedly.
When we view people and work in these terms, we can learn a great deal about leading from Netflix. In fact, when I learnt their recommender equation, it instantly made sense to me.
Imagine that the overall average rating for movies is 3.7. With this as a baseline – if ‘Rocky’ has an average rating of 3.9 – then the movie offset is 0.2. Then, if a viewer’s average rating of movies is 3.6, the viewer offset is -0.1. In this case, the baseline rating is 3.8 (3.7 + 0.2 – 0.1).
We need to break down work in the same way.
There is an average productivity and performance for work, then there is an offset depending on the task (work completed) and, more specifically, on the trigger (what causes the work to be done).
Just as Netflix uses its offset data to make recommendations to its subscribers, breaking work down in this way gives you a deeper understanding of how to maximise performance and productivity.
Why not use this model to create your own personalised recommender? By understanding the offset, you can become more specific in your leadership nudges and actions.
Business