Rapid or Right? Making Startup Decisions Like Scientists
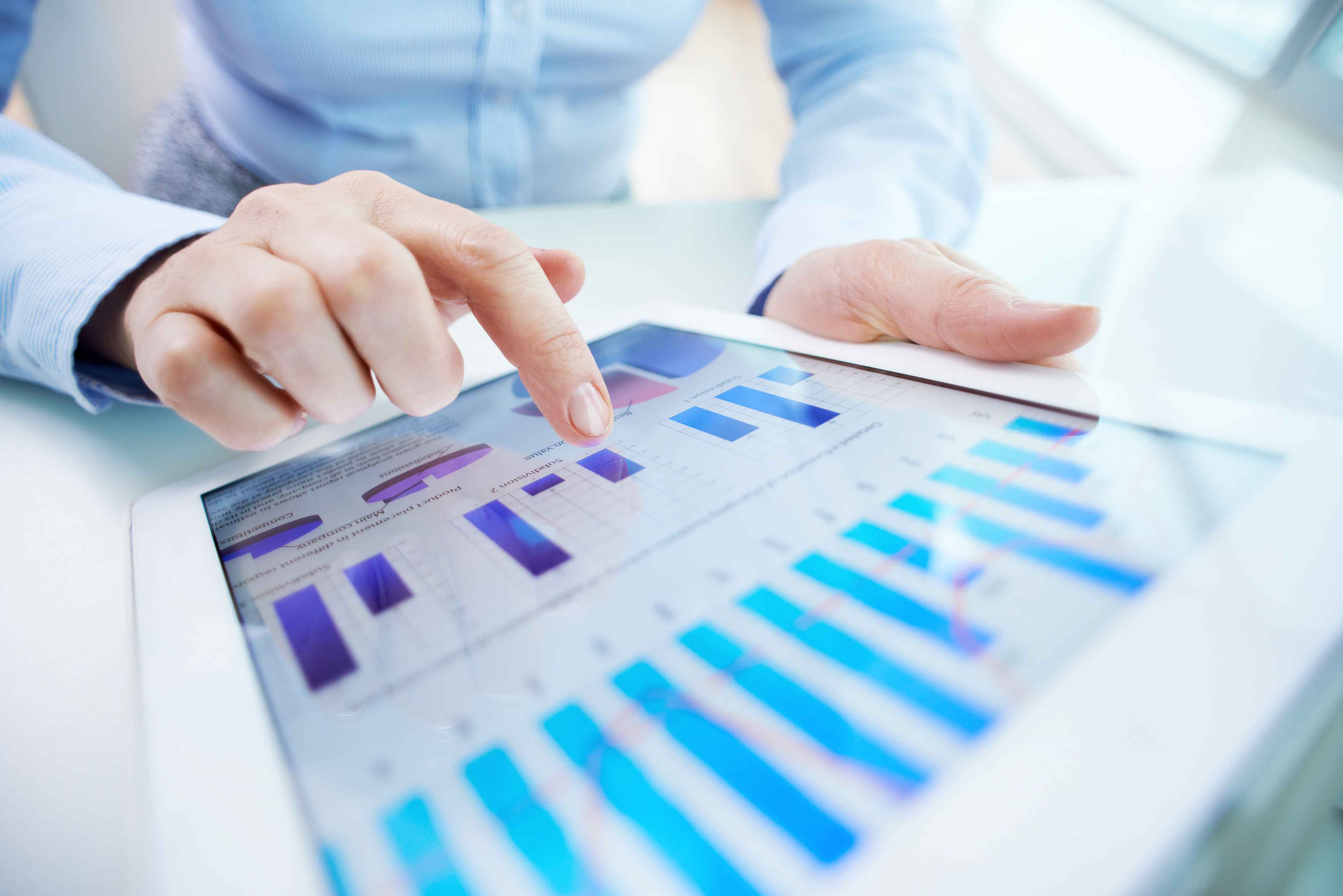
Pressfoto from Freepik
The mantra "make data-driven decisions" has become gospel in entrepreneurial circles. In recent years, scientific methods – forming hypotheses, gathering evidence and letting data guide decisions – have gained traction as a way to improve startup outcomes.
However, our recent research, published in the Strategic Management Journal and featured in Harvard Business Review, turns up an important caveat: The scientific approach can hamper the short-term economic performance of some startups, particularly those in their infancy.
Existential questions
We conducted a field experiment involving 261 startups across various industries and development stages in the United Kingdom. All participating entrepreneurs received training in decision-making frameworks, such as the business model canvas, and skills like A/B testing. Half were additionally trained in scientific decision-making approaches.
We assessed each company's business model maturity based on their openness to pivotal changes. Through regular interviews and nine months of revenue tracking, we uncovered a critical pattern: Mature startups primarily applied scientific methods to optimise existing strategies, while early-stage ventures used them to question fundamental business assumptions.
Optimisation vs. exploration
For mature startups, scientific decision-making meant fine-tuning what was already working. One participant who founded a business that sold memory cards and other electronics products described using structured experimentation to test different product descriptions.
“We started advertising our products as…memory solutions for those specific devices,” the founder told us. “Once we had these different listings… we [found this to be] the best method.” The company increased profits by 10 percent after changing its approach to product listings.
Contrast this with early-stage founders, who tended to apply scientific rigour to more existential questions. “Before coming into the programme (experiment), we had a very clear vision,” said one participant. “But as we rigorously tested our hypotheses and ideas, we’re less clear on which pain points we’re reducing for users. So that’s the thing we’re [now] trying to ensure.”
This deeper questioning often led to significant strategic shifts that temporarily slowed growth while founders relooked their entire business models.
Short-term pain, long-term gain?
A limitation of our research was the relatively brief nine-month measurement window. While early-stage startups saw revenues decline after implementing scientific decision-making, many founders viewed this period positively, believing their exploratory work would drive stronger long-term outcomes.
"We've taken a step back to reevaluate everything," one early-stage founder reflected. "It feels necessary for building something truly sustainable rather than chasing quick wins."
Another mentioned they were "sacrificing immediate growth to establish a genuinely viable long-term business model."
We hypothesise that the uncertainty in this initial phase, though challenging for metrics-focused stakeholders, may ultimately drive superior long-term performance. By thoroughly examining core assumptions before making major commitments, early-stage ventures might avoid the trap of achieving short-term growth at the expense of long-term viability.
Why quality matters more than speed in the long run:
Strategic recommendations by growth stage
For early-stage founders
Embrace uncertainty as a natural part of exploration. Prepare for potential short-term performance dips while thoroughly investigating fundamental assumptions. Focus on developing a robust "theory of value" before optimising metrics. Most importantly, communicate clearly with stakeholders about this exploratory phase – rushing through it could put your venture on a path to eventual failure despite initial growth.
For established startup leaders
Apply scientific methods to optimise established business framework. Implement systematic testing across product features, marketing approaches and operational processes. Rigorously track the impact of these incremental improvements to capitalise on what's already working.
For investors
Adjust performance expectations based on business model maturity. Value thorough strategic exploration in early stages, even when it temporarily impacts growth metrics. Rather than focusing exclusively on immediate results, look for evidence of systematic learning within the organisation – this often signals stronger long-term potential.
Using the right tool at the right time
The key insight of our study isn't that scientific decision-making helps or hurts certain companies – it's that timing and context are crucial. Early-stage ventures need space to explore and question fundamental assumptions, even when that creates temporary uncertainty. More established companies can apply scientific methods to optimise existing frameworks, generating more immediate performance improvements.
Successful founders must understand this distinction and adapt accordingly. There's a time to step back and reassess fundamentals, and a time to apply rigorous testing to refine what's working. Scientific decision-making resembles any sophisticated tool – its impact depends not just on whether you use it, but on what problems you apply it to.
The scientific approach remains valuable throughout a startup's journey, but how we measure its success must evolve as the business matures. By matching the right decision-making approach to their firm’s current stage of development, entrepreneurs can maximise their chances of both short-term survival and long-term success.
Join 500+ C-suite leaders as they explore cutting-edge insights in leadership, transformation, and growth. Reserve your spot at the Malaysia Leadership Summit 2025 now!.
Edited by: Seok Hwai Lee
Business
Chiara Spina is an Assistant Professor of Entrepreneurship at INSEAD. Her research focuses on understanding how entrepreneurial firms leverage systematic decision-making and experimentation to innovate and grow.
Elena is a Professor of Strategy at Bayes Business School, City St George's, University of London. She holds a Ph.D. and M.Sc. cum laude in Business Administration from Bocconi University, Milan.